The long-standing effort to manage enterprise knowledge has repeatedly failed due to the inability...
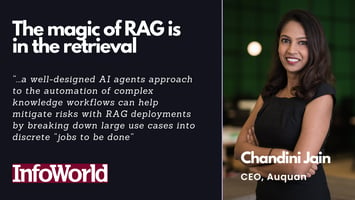
A process called retrieval-augmented generation (RAG) is unlocking the kinds of enterprise generative AI use cases that previously were not viable.
Auquan CEO Chandini Jain has a new byline in InfoWorld: How RAG completes the generative AI puzzle.
In her article, Chandini explores what RAG is and how it works to the limitations of LLMs and how to overcome them.
In the past year, generative AI has been a hot topic of conversation for transforming workflows in nearly every industry, but implementations have not been successful for complex knowledge-intensive enterprise use cases.
That’s because the current state of generative AI has limitations that prevent success in the enterprise, including hallucinations, a lack of domain-specific, and up-to-date data.
In order to address these limitations, Retrieval Augmentation Generation (RAG) combines a retrieval model with a generative model in order to enable LLMs to access external data. This helps ensure enterprises get timely, trustworthy, and transparent data.
“RAG brings to generative AI the one big thing that was holding it back in the enterprise: an information retrieval model. Now, generative AI tools have a way to access relevant data that is external to the data the large language model (LLM) was trained on—and they can generate output based on that information. This enhancement sounds simple, but it’s the key that unlocks the potential of generative AI tools for enterprise use cases.”
Read Chandini’s full byline in InfoWorld.
Each day we spotlight under-the-radar investment themes and idiosyncratic risks pulled from our intelligence engine, often involving emerging markets, supply chain issues, ESG risks, and the impact of regulatory changes.
The long-standing effort to manage enterprise knowledge has repeatedly failed due to the inability...
The financial services industry is knowledge-intensive, requiring professionals to constantly...
Auquan was named to the second annual edition of the ESGFinTech100, which was released today by...
Each day we spotlight under-the-radar investment themes and idiosyncratic risks pulled from our intelligence engine, often involving emerging markets, supply chain issues, ESG risks, and the impact of regulatory changes.
Interested in working at Auquan? Click Here